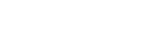
Stop-loss adjusted labels for machine learning-based trading of risky assets (Financ. Res. Lett.), Prof. Dong-Young Lim
Stop-loss adjusted labels for machine learning-based trading of risky assets
Author: Yoontae Hwang , Junpyo Park , Yongjae Lee , Dong-Young Lim
Since the rise of ML/AI, many researchers and practitioners have been trying to predict future stock price movements. In actual implementations, however, stop-loss is widely adopted to manage risks, which sells an asset if its price goes below a predetermined level. Hence, some buy signals from prediction models could be wasted if stop-loss is triggered. In this study, we propose a stop-loss adjusted labeling scheme to reduce the discrepancy between prediction and decision making. It can be easily incorporated to any ML/AI prediction models. Experimental results on U.S. futures and cryptocurrencies show that this simple tweak significantly reduces risk.