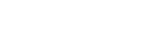
Stereo Object Matching Network(Prof. Kyungdon Joo)
저 자 | {Jaesung Choe, Kyungdon Joo}*, Francois Rameau, and In So Kweon |
---|---|
학 회 | IEEE International Conference on Robotics and Automation (ICRA) |
논문일시(Year) | 2021 |
논문일시(Month) | 06 |
Abstract
This paper presents a stereo object matching method that exploits both 2D contextual information from images as well as 3D object-level information. Unlike existing stereo matching methods that exclusively focus on the pixel-level correspondence between stereo images within a volumetric space (i.e., cost volume), we exploit this volumetric structure in a different manner. The cost volume explicitly encompasses 3D information along its disparity axis, therefore it is a privileged structure that can encapsulate the 3D contextual information from objects. However, it is not straightforward since the disparity values map the 3D metric space in a non-linear fashion. Thus, we present two novel strategies to handle 3D objectness in the cost volume space: selective sampling (RoISelect) and 2D-3D fusion (fusion-by-occupancy), which allow us to seamlessly incorporate 3D object-level information and achieve accurate depth performance near the object boundary regions. Our depth estimation achieves competitive performance in the KITTI dataset and the Virtual-KITTI 2.0 dataset.