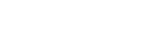
POEM: Polarization of Embeddings for Domain-Invariant Representations (AAAI 2023), Prof. Sung Whan Yoon
Handling out-of-distribution samples is a long-lasting challenge for deep visual models. In particular, domain generalization (DG) is one of the most relevant tasks that aims to train a model with a generalization capability on novel domains. Most existing DG approaches share the same philosophy to minimize the discrepancy between domains by finding the domain-invariant representations. On the contrary, our proposed method called POEM acquires a strong DG capability by learning domain-invariant and domain-specific representations and polarizing them. Specifically, POEM cotrains category-classifying and domain-classifying embeddings while regularizing them to be orthogonal via minimizing the cosine-similarity between their features, i.e., the polarization of embeddings. The clear separation of embeddings suppresses domain-specific features in the domain-invariant embeddings. The concept of POEM shows a unique direction to enhance the domain robustness of representations that brings considerable and consistent performance gains when combined with existing DG methods. Extensive simulation results in popular DG benchmarks with the PACS, VLCS, OfficeHome, TerraIncognita, and DomainNet datasets show that POEM indeed facilitates the category-classifying embedding to be more domain-invariant.