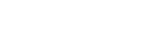
Quantifying incident impacts and identifying influential features in urban traffic networks (Transportmetrica B-Transp. Dyn.), Prof. Sungil Kim
Traffic incidents are a common occurrence in urban traffic networks, but predicting their impacts is challenging because of network complexity and the dynamic spatial and temporal dependencies inherent in traffic data. Nevertheless, the prediction of traffic incident impacts is crucial for global positioning systems to provide drivers with real-time route recommendations for bypassing congested roads. To this end, we formulated nonrecurrent congestion measures to quantify these impacts and developed a new method to identify the influential features that locally affect individual incidents. Because traffic incident impacts are determined by a complex entanglement of local features, a meaningful feature that can explain their impacts globally may not exist. Consequently, to identify all influential local features, we applied the local interpretable model-agnostic explanations (LIME) technique to the proposed nonrecurrent congestion measures. The proposed method was validated using real user trajectory data and incident data provided by the NAVER Corporation and the Korean National Police Agency, respectively.