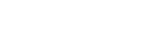
MMP Net: A feedforward neural network model with sequential inputs for representing continuous multistage manufacturing processes without intermediate outputs (IISE Trans.), Prof. Chiehyeon Lim
Abstract
Machine learning models that are used for the prediction and control of production can improve quality and yield. However, developing models that are highly accurate and reflective of real-world processes is challenging. We propose a feedforward neural network model specifically designed for continuous multistage manufacturing processes (MMPs) without intermediate outputs. This model, which is termed “MMP Net,” can accurately represent the control mechanism of continuous MMPs. Whereas existing studies on learning MMPs assume an intermediate output data, the MMP Net does not require such an unrealistic assumption. We use the MMP Net to develop prediction models for the lubricant base oil production process of a world-leading lubricant manufacturer. Evaluation results show that the MMP Net is superior to other deep neural network and machine learning models. Consequently, the MMP Net is actually implemented in the real factory in 2022 and is expected to save 0.9 million dollars per year for each production line. We believe that our work can serve as a basis to develop customized machine learning solutions for improving continuous MMPs.